INTRODUCTION
Explore the interesting terrain of AI, where sophisticated technology meets everyday application.
It’s unbelievable how AI is affecting virtually every business you can imagine.
And we’re seeing it all from the way we buy things online to the new self-driving car technology.
While algorithms and apps get the attention, a crucial shift in the hardware powering AI is happening quietly, and that’s our focus.
We’re discussing this enormous demand that’s developing so quickly for bespoke semiconductor chips, all fueled by the escalating complexity of AI reasoning.
For this deep dive, we’ll distill the essential takeaways and explain why this shift to custom AI silicon is crucial for staying updated on AI trends.
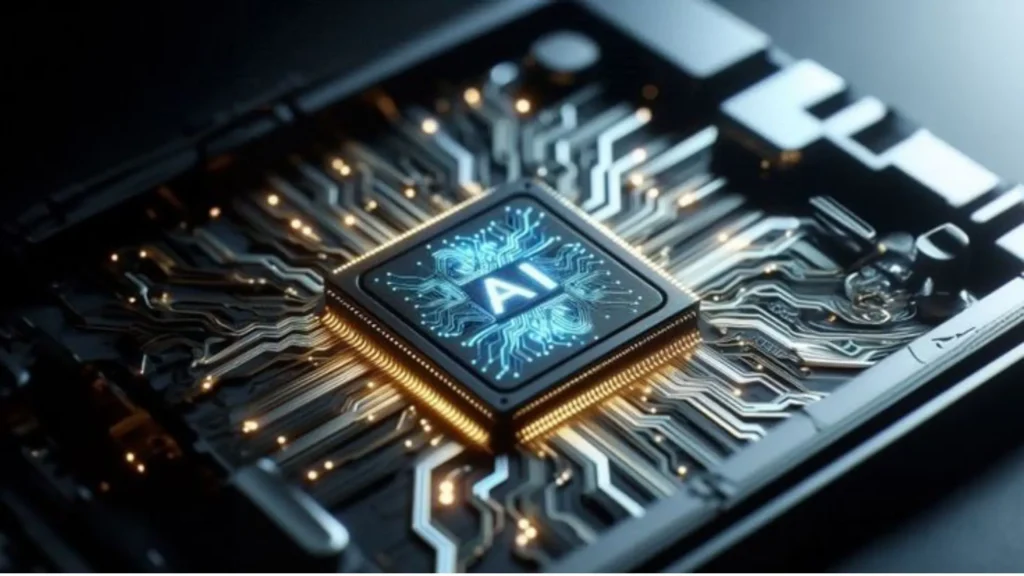
When we’re discussing AI reasoning, what does that signify?
Because it does sound like I have no idea. It’s something beyond a simple program doing some arithmetic.
AI has long done pattern recognition, but the exciting shift now is to be reasoning, which this article explores.
It’s decision making, logic, and having a sense of context.
You know, not just recognizing an image as a dog, it’s knowing what a dog is, how a dog usually behaves, like, even if it’s happy or playful based on little things.
And all that takes a whole different amount of computational power.
It’s almost like AI is gaining some sort of deeper knowledge. And those are becoming so advanced.
And then you’ve got these multimodal AI systems that are processing text, images, audio, all simultaneously.
It’s amazing to consider all that information.
According to the article, real-time, human-quality AI output requires massive processing power to combine information, not just locate it.
Picking up on those nuances and then being able to form these, you know, sensible answers that make sense within the context of whatever is occurring.
So we’re not necessarily talking about just these chatbots here. I mean, things like self-driving cars Right.
Matrix Calculations and Parallel Processing: The Driving Force Behind Custom AI Silicon
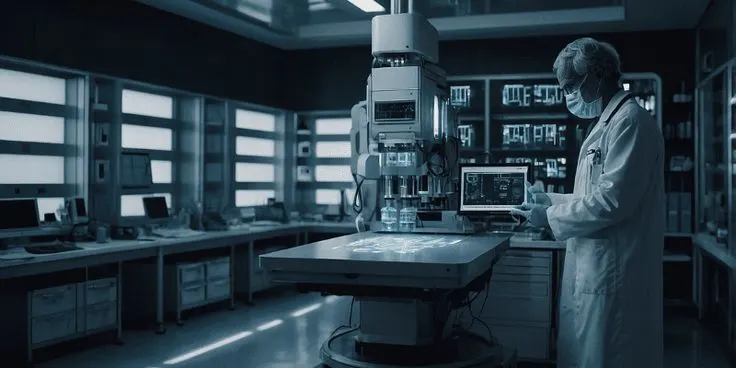
Gigantic leaps in health care, even robotics.
I can’t even conceive of the level of logic a self-driving car must use to drive safely, say, through a busy intersection or for an AI to assist with some sort of complicated medical diagnosis.
And when you consider the larger picture, these sophisticated AI applications, they’re not just performing these simple calculations.
They’re always processing these huge data sets, predicting things, and they must respond to these changing situations that are happening quickly.
So that requires a whole different kind of computation than what we’ve applied to traditional software.
So, I suppose your typical CPU, the central processing unit that’s in most computers, just isn’t built for this type of load.
And the article even refers to these high dimensional matrix calculations.
Matrix calculations are like simultaneously figuring out how hundreds or thousands of factors relate to make a prediction, just on a grand scale.
AI also requires super high-speed memory access and the capacity for millions/billions of simultaneous operations – parallel processing on a huge scale.
It’s kind of like having this enormous army of workers, each of them working on a different aspect of a gigantic complex puzzle.
And they’re all running simultaneously. So that’s where the article jumps to this compelling concept.
This need for efficiency is what is driving this entire transition to custom silicon.
So, what does that entail, exactly? I mean, are we talking about, like, creating chips from scratch? That’s basically it.
This brings up this significant question about how we design the fundamental building blocks that drive AI systems.
So custom silicon essentially refers to designing chips that are specifically designed and optimized for those specific AI tasks.
Google’s TPUs and the Rise of Application-Specific AI Hardware
The article divides it into a couple of broad categories beginning with ASICs.
So that’s application specific integrated circuits.
So, is it more like having these specialized tools for a very specialized job?
ASICS are designed from the ground up for AI workloads.
And that’s why they might be able to go at much higher speeds and be considerably more energy-efficient than those generic purpose chips.
They’re discussing Google’s tensor processing units, which are the TPUs.
The TPUs. And those are specifically tuned for the extremely intensive calculations that are required for AI inference.
So that’s really when you have a trained AI model that is predicting on new data.
But they’re also used to train those models in the first place.
It’s like having a dedicated kitchen appliance that’s far more efficient for its specific purpose than a general one.
This trend towards task specific hardware is sort of a reflection of how specialized biological structures have developed for specific purposes.
So it appears there’s a similar concept at work when we’re discussing computational efficiency.
That’s interesting. So ASICs are all about performance maximization for a given set of tasks.
And then the article discusses FPGAs. So FPGAs or field programmable gate arrays, they provide something a bit unique, flexibility.
What’s great about them is that they can be reprogrammed.
Flexible and Reprogrammable Hardware for Dynamic AI Workloads
Unlike ASICs, which are sort of cast in concrete, FPGAs can be programmed differently after they’re produced.
So the article mentions Microsoft’s Azure AI as a great place where these FPGAs are being utilized for real time AI.
And this versatility makes them extremely handy for AI models that are always being revised and are changing constantly.
It’s kind of like having a piece of hardware that can change what it does based on what the AI software requires.
GPUs were initially created to render those complex graphics.
Their parallel processing is well-adapted to the large matrix computations critical for cutting-edge AI.
NVIDIA’s Dominance, the Rise of Neuromorphic Chips, and Cloud Providers’ Custom AI Push
And the article highlights that NVIDIA has been leading in this field for years, particularly with their architectures such as the h 100 and their latest b series GPUs.
So in a way, GPAs sort of accidentally ended up as these AI horses, and then they’re designed to do just this now.
And then the final form of custom silicon that the article discusses is neuromorphic chips. They are certainly cutting edge.
Neuromorphic chips mimic the human brain and are promising for efficient, low-power AI inference, making them great for edge computing.
So you imagine a smart sensor, a small robot that must decide very quickly without having to continuously communicate with a server somewhere distant in the cloud.
Neuromorphic chips would truly be a game changer.
So, we’ve got all these various forms of custom silicon surfacing, and it’s most certainly not a one size fits all anymore.
And then there’s the follow-up in how these large cloud providers are also jumping into creating their own chips.
Those big players like AWS, Google Cloud, Microsoft Azure, they’re investing a lot of money into creating their own AI chips internally. So what’s behind it?
Is it simply cost, or is there another factor? Well, there are a couple of reasons why. First, they want to get the absolute optimal performance out of their AI services.
They want to fine tune all of that for their own hardware and make it as efficient as possible.
AWS Leads the Charge: Developing Custom AI Chips for Performance, Cost, and Sustainability
Investing in custom chips reduces reliance on traditional manufacturers, giving them greater control over costs, speed of innovation, and overall liberty.
And I think the article does have some quite interesting examples of this. Such as, AWS has come up with its own chips for artificial intelligence.
They have Trainum, which is optimized for training AI models that do computationally heavy lifting.
And then they have Inferential, which is optimized for that efficient AI inference we were discussing.
And they want to be able to provide their customers with a cheaper option than those high-end GPUs that have kind of taken over AI processing in the cloud.
Creating the fastest, most efficient AI infrastructure comes with a challenge: the huge energy demands won’t be cheap to operate.
It’s a very valid observation on the part of the article.
Data centers, where all this computing hardware for cloud-based AI resides, they consume a lot of electricity.
So it’s extremely critical to design those chips with better performance per watt, do more with the same energy.
That’s how you do AI not only more capable, but also sustainable. And environmentally friendly.
What’s the future of AI chips?
Because the article suggests some seriously crazy things in the pipeline.
The future of AI chip technology is exciting. There are a few main areas that the article brings up. One is this concept of three d chip stacking.
it’s stacking chips in a 3D configuration to significantly improve data access and processing for intensive AI tasks. Think of it as building upwards.
It’s like making more room within a smaller footprint. That’s such a good way to describe it.
So, what else is in the pipeline? Well, there’s photonics-based AI chips Photonics based?
And this is about computing using light rather than electricity.
The possibilities there are amazing. I mean, you could have AI processing speeds that are unprecedented because light moves much faster than electrons. So are we saying, like, rather than copper wiring, it’s more like fiber optics for computation. That’s a great analogy.
And then, sure, there’s quantum computing. The article talks about quantum AI accelerators It’s still very early stage, though.
Research is underway on quantum computers, which theoretically could solve today’s most complex, unsolvable problems.
So, it’s a longer-term project, but it could be revolutionary for certain types of AI challenges.
It’s like the next frontier. And then, of course, there are edge AI chips.
We touched on that a bit with those neuromorphic designs, but it’s a much larger movement.
The Custom Silicon Revolution: Powering the Next Era of AI
Edge AI is all about taking AI processing right to the location of the data. So, consider all those intelligent devices, robots, sensors, and the entire Internet of things.
And its specialized chips for those uses that are important.
Because you want that real time processing without the lag of sending all the data up to the cloud and back.
So, the general takeaway from this article appears to be that the semiconductor industry is just in this state of continual innovation.
And it’s all being fueled by these constantly escalating requirements of AI reasoning.
It’s not just a matter of, you know, shrinking chips anymore. It’s about rethinking the entire design, the materials, everything.
Investing in custom AI silicon will give companies a huge advantage in the expanding AI economy, as hardware is as vital as algorithms.
It’s this massive technological revolution where the silicon is a foundational part of the puzzle.
Massive Demand for Custom Silicon: A Paradigm Shift in the AI Era
So, if we’re going to summarize our dive into this AI World Journal article, I think the bottom line is that there’s just massive increasing demand for custom silicon.
It’s not a blip on the screen. It’s a paradigm change that’s shifting the future of AI and the semiconductor industry at large.
I couldn’t agree more. And we’ve spoken about how sophisticated AI reasoning is becoming.
It’s not all about pattern recognition anymore. It’s about complicated decision making and context understanding.
And because of that, we require these application-specific hardware solutions. Those standard CPUs and even the general-purpose GPUs, they’re just insufficient.
And this is why we’re witnessing such incredible innovation with ASICs, FPGAs, neuromorphic chips, and those cloud providers creating their own chips internally.
The incredible rate at which AI is advancing fills one with wonder about the next generation of hardware and its potential to alter our lives in ways we cannot yet grasp.
The way these algorithms and the physical infrastructure work together to make them real is truly astonishing.